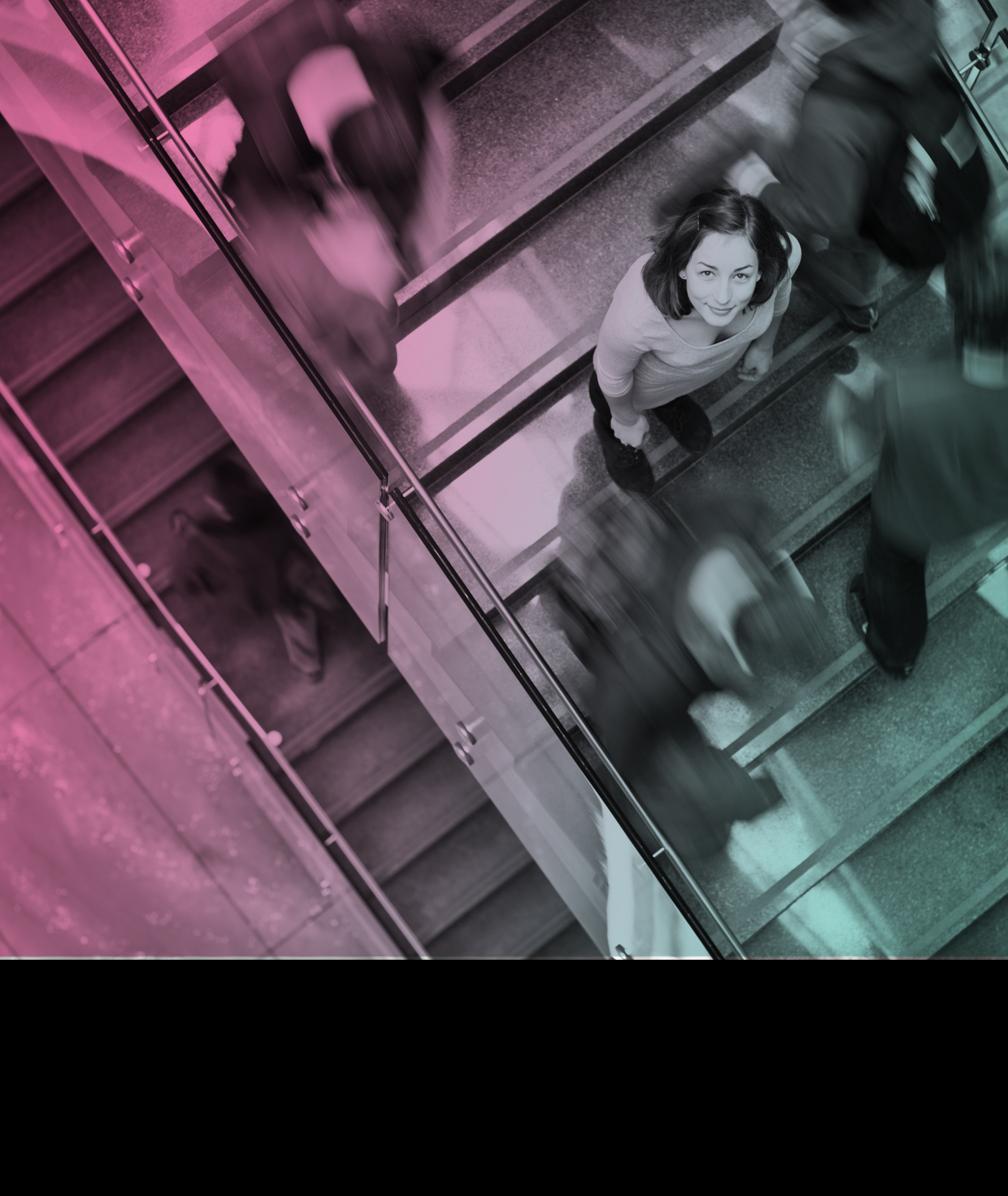
Unlocking AI Success: The Overlooked Importance of Evaluation Data
24 Feb 2025 | Loghman Zadeh
Unlocking AI Success: The Overlooked Importance of Evaluation Data
The Intersection of AI Evaluation and Business Goals
AI Success Pyramid
The Missing Piece: Evaluation Data
When organizations embark on AI initiatives, they often focus on acquiring large datasets for the problem at hand. However, they frequently overlook a critical question: How will we measure success? Evaluation data—datasets specifically designed to test AI outputs against ground truth or business goals—is essential for ensuring AI systems function as expected. Despite its importance, many enterprises lack the internal processes to generate or collect meaningful evaluation data before starting an AI project. This gap leads to prolonged development cycles, poor model performance, and, ultimately, AI systems that don’t meet strategic objectives.
Why Evaluation Data Matters
- Measuring System Performance – AI systems need structured benchmarks to gauge their accuracy, relevance, and reliability. Without evaluation data, it’s impossible to determine if an AI-powered solution is improving business outcomes or simply producing impressive but ineffective outputs.
- Reducing Business Risk – Deploying an AI system without proper evaluation is like launching a product without user testing. Poor AI performance can lead to misinformed decisions, operational inefficiencies, and reputational risks.
- Optimizing AI Systems Over Time – AI is not a “set-and-forget” solution. Continuous monitoring and improvement require well-defined metrics, which can only be established with a robust evaluation dataset.
How Enterprises Can Prepare for AI Projects
To maximize the impact of AI initiatives, businesses should take proactive steps to build an evaluation data strategy:
- Define Success Metrics Early: AI should be evaluated based on business objectives, whether it’s accuracy, efficiency, cost savings, or user satisfaction.
- Establish Data Collection Processes: Enterprises should integrate evaluation data collection into their existing workflows, ensuring ongoing measurement and refinement of AI performance.
- Work with AI Experts: Partnering with experienced AI providers can help define what good evaluation data looks like and how to gather it effectively.
Evaluation Data for Retrieval-Augmented Generation (RAG) Systems
- Question-Answer Pairs (QA Pairs): A dataset comprising questions and their corresponding answers is fundamental. These pairs should be derived from the knowledge base or documents that the RAG system utilizes. This ensures that the evaluation reflects the system’s ability to retrieve and generate accurate information based on its training data.
- Document Relevance Annotations: Each question-answer pair should be associated with annotations indicating the relevance of the retrieved documents. This allows for the assessment of the retrieval component’s effectiveness in sourcing pertinent information.
- Generation Quality Metrics: In addition to retrieval accuracy, evaluating the quality of the generated responses is crucial. Metrics such as coherence, grammatical correctness, and contextual relevance are important for assessing the generative aspect of the system.
Evaluation Data for Agentic AI Solutions
- Task-Specific Scenarios: Datasets should include a variety of scenarios that the AI agent is expected to handle. These scenarios should encompass the range of tasks the agent will perform, providing a comprehensive basis for evaluation.
- Performance Metrics: Data should be annotated with performance metrics relevant to the tasks, such as accuracy, efficiency, and error rates. This enables the assessment of the agent’s effectiveness in achieving desired outcomes.
- Behavioural Annotations: Annotations that capture the agent’s decision-making process, including reasoning and actions taken, are valuable. These annotations help understand the agent’s behaviour and ensure alignment with intended objectives.
- Real-Time Performance Data: For agents operating in dynamic environments, real-time performance data is essential. This data should reflect the agent’s performance under various conditions, including latency and adaptability to changing inputs.